|
 |
Printable
version |
The NCC Results (May 2009 - May 2010)
The CINBAD (CERN Investigation of Network
Behaviour and Anomaly Detection) project was
launched in 2007 in collaboration with HP ProCurve.
This challenging project’s mission is to understand
the behaviour of large computer networks in the
context of high-performance computing and campus
installations such as CERN. CERN’s campus network
has more than 50 000 active user devices
interconnected by 10 000 km of cables and fibres,
with more than 2500 switches and routers. The
potential 4.8 terabit per second throughput within
the network core and 140 gigabit per second
connectivity to external networks offer countless
possibilities to different network applications. The
CINBAD goals are to detect traffic anomalies in such
systems, perform trend analysis, automatically take
counter-measures and provide post-mortem analysis
facilities. The project is divided into three
phases: data collection and network management, data
analysis and algorithm development, performance and
scalability analysis. This research activity is now
producing practical results as well as providing
crucial information to the CERN security team.
Project
The starting point of the project was to define
the requirements and ensure a common framework of
precise definitions, for example, what constitutes
an anomaly or a trend. The following common
denominator emerged: an anomaly is always a
deviation of the system from the normal (expected)
behaviour (baseline); the normal behaviour
(baseline) is never stationary and anomalies are not
always easy to define. As a consequence, anomalies
are not easy to detect. However, some potential
anomaly detectors can be identified. Thus the use of
statistical detection methods can be considered. By
learning the ‘normal behaviour’ from network
measurements, and continuously updating the ‘normal
baseline’, it is possible to detect new, unknown
anomalies. Applying such a method also has some
drawbacks as it is still possible to attempt to
force a false negative, the selection of suitable
input variables is an issue (many anomalies being
within ‘normal’ bounds of the metrics), and finally
a false positive can be extremely costly and does
not provide a satisfactory anomaly type
identification.
With the modern high-speed networks it is
impossible to monitor all the packets traversing the
links. sFlow is the industry standard for monitoring
computer networks by means of random packet
sampling. In fact sFlow is derived from the
collaboration between HP, the University of Geneva
and CERN in 1991. The team decided that the best way
to monitor the network on a large scale was to use
data statistical analysis by packet sampling. In the
first phase of the project the CINBAD team
investigated the feasibility of packet sampling in
the context of anomaly detection. The results of
this research were published at the end of 2007 in a
report ‘Packet Sampling for Network Monitoring’, by
Milosz Hulbój and Ryszard Jurga.
These studies, complemented by an
in-depth analysis of the sFlow agents, enabled the
CINBAD team to design and implement the sFlow data
collector. Given the huge amount of sFlow data (300
000 samples per second) to be collected and analysed,
the team decided to benefit from CERN’s know-how in
data storage and analysis. During the survey on data
acquisition, the LHC experiments and Oracle experts
were consulted on high-performance data storage,
data format and representation and analysis
principles. This survey led the team to the design
of the multi-stage sFlow collector (see figure
below) and to implement it. Since summer 2008, the
collection system has been successfully running on a
large-scale network, using approximately 1000 HP
switches.
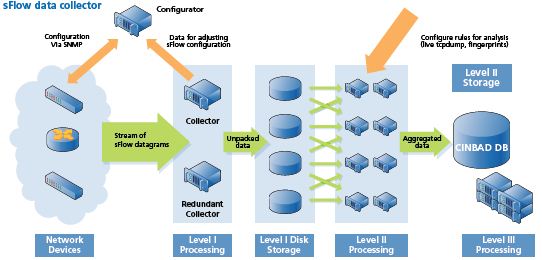
Results
The team developed tools for
analysing the stored data based on these data
collection tests results. Various data analysis
techniques have been tested, among them a
statistical data analysis and time series mining and
signature based approach. In addition to these
tools, the team adapted SNORT (open source network
intrusion prevention and detection system) to work
with sampled sFlow data. This SNORT setup was
complemented by open source traffic rules as well as
in-house CINBAD rules. Initial data analysis has
enabled the team to detect some misbehaviour and a
certain number of anomalies in the CERN network. It
appears that most of these security anomalies
(malicious software, policy violations) originated
from end-user machines.
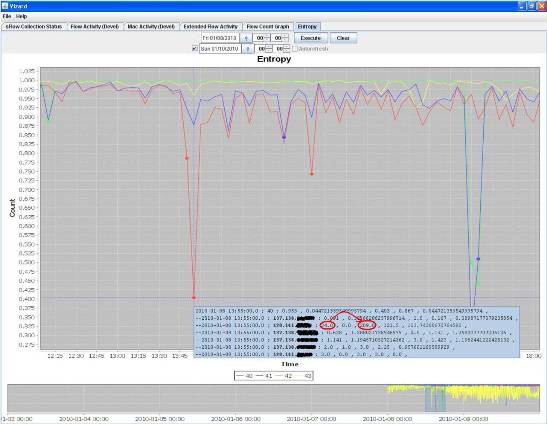
CINBAD tools allow for easy identification of
the anomalous hosts via analysis of the network
parameters’ entropy.
Over the past few years, the CINBAD team has
collected and analysed more than 30 terabytes of
data in order to investigate various approaches to
enhancements for Network Monitoring and Anomaly
Detection. Now, the CINBAD project is turning into
the production stage and is delivering more and more
new facilities. The current CINBAD toolkit (C-Eye)
enables one to visualise detailed information on the
actual state of the network at any point in time.
This new facility improves day-to-day network
operations as well as reducing the time needed to
diagnose network problems, since detailed
information about the activity of each machine is
available. The trend analysis tool provides metrics
about evolution of the CERN network. Information
about the number of active devices, network
infrastructure usage, protocol distribution as well
as other network statistics constitutes valuable
input to the future design. In addition, the team is
delivering a tool that enables one to browse the
alerts from the CINBAD anomaly detection engine as
well as identify their causes. All the
aforementioned tools have already proved to be
useful in resolving problems, including printer
abusers, spammers and non-legitimate network scans.
However, even though the project is entering the
final stage, the team is continuously searching for,
and identifying, new enhancement possibilities.
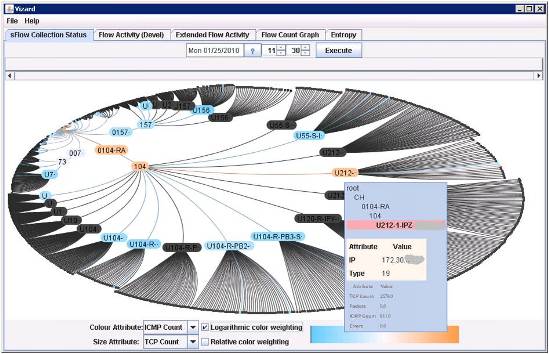
CINBAD
tools for network operation (C-Eye) are being
currently integrated into the network management
platform at CERN.
At the end of March, the CINBAD team visited the
HP ProCurve group in Roseville, USA. The main goal
of this visit was to present and discuss the latest
results and transfer the knowledge that was gathered
during the course of the project. CINBAD ideas,
tools and algorithms were very well received by
people from different groups of ProCurve. HP is
considering integrating some of them into their
products. This, together with the ongoing deployment
and integration at CERN is clear evidence of the
project’s success.
Since February 2010, a
new openlab team under the
codename WIND (Wireless
Infrastructure Network
Deployment) carries out
research and provides new
algorithms, guidelines and
solutions to support the
deployment and operation of the
Wi-Fi infrastructure at CERN.
More information on this new project are
available in the WIND
section.
|
|
|
|